Yan Scholten
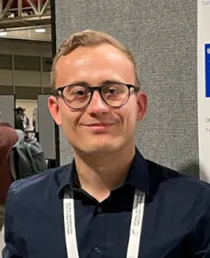
Technical University of Munich
Department of Computer Science - I26
Boltzmannstr. 3
85748 Garching b. München
Germany
Room: 00.11.065
E-Mail: y.scholten [at] tum.de
Website: yascho.github.io
Research Interests
- Reliable machine learning
- Conformal prediction
- Machine unlearning
- Machine learning on graphs
Selected Publications
Full list on Google Scholar
- Provably Reliable Conformal Prediction Sets in the Presence of Data Poisoning
Yan Scholten, Stephan Günnemann
International Conference on Learning Representations, ICLR 2025 (Spotlight)
[PDF | Project page | Code]
- A Probabilistic Perspective on Unlearning and Alignment for Large Language Models
Yan Scholten, Stephan Günnemann, Leo Schwinn
International Conference on Learning Representations, ICLR 2025 (Oral)
[PDF | Project page | LLM framework (Code) | Confidence bounds (Code)]
- Adversarial Alignment for LLMs Requires Simpler, Reproducible, and More Measurable Objectives
Leo Schwinn, Yan Scholten, Tom Wollschläger, Sophie Xhonneux, Stephen Casper,
Stephan Günnemann, Gauthier Gidel
[Preprint], 2025.
- Hierarchical Randomized Smoothing
Yan Scholten, Jan Schuchardt, Aleksandar Bojchevski, Stephan Günnemann
Advances in Neural Information Processing Systems (NeurIPS), 2023
[PDF | Project page | Code]
- (Provable) Adversarial Robustness for Group Equivariant Tasks:
Graphs, Point Clouds, Molecules, and More
Jan Schuchardt, Yan Scholten, Stephan Günnemann
Advances in Neural Information Processing Systems (NeurIPS), 2023
[PDF | Project page | Code]
- Randomized Message-Interception Smoothing: Gray-box Certificates for Graph Neural Networks
Yan Scholten, Jan Schuchardt, Simon Geisler, Aleksandar Bojchevski, Stephan Günnemann
Advances in Neural Information Processing Systems (NeurIPS), 2022
[ PDF | Project page | Code ]
Education
- 2022-now: PhD student in Computer Science, Technical University of Munich
- 2019-2022: M.Sc. Informatics - Technical University of Munich (passed with high distinction)
- 2015-2019: B.Sc. Computer Science (Math Minor) - Paderborn University (passed with distinction)
Academic Honors and Awards
- 2023: Admission to the Konrad Zuse School of Excellence in Reliable AI
- 2019: Deutschlandstipendium awarded by the Technical University of Munich
- 2018: RISE worldwide scholarship awarded by DAAD
- 2018: Deutschlandstipendium awarded by Studienfonds OWL
- 2017: Admission to elite program of the EIM-faculty at Paderborn University