Jan Schuchardt
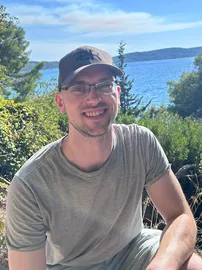
Technical University of Munich
Department of Informatics - I26
Boltzmannstr. 3
85748 Garching b. München
Germany
Room: 00.11.061
Phone: +49 (0)89 / 289-17380
E-Mail: j.schuchardt [at] tum.de
GitHub: jan-schuchardt
Research Focus
My research is focused on making machine learning models for graphs, images, sequences, and other structured data more trustworthy. Specifically, I am interested in methods that provably guarantee robustness to data perturbations at training and inference time.
Publications
Privacy Amplification by Structured Subsampling for Deep Differentially Private Time Series Forecasting
(selected for spotlight presentation)
Jan Schuchardt, Mina Dalirrooyfard, Jed Guzelkabaagac, Anderson Schneider, Yuriy Nevmyvaka, Stephan Günnemann
International Conference on Machine Learning (ICML), 2025
Also presented at Workshop on Advances in Financial AI (ICLR), 2025
[PDF]
Unified Mechanism-Specific Amplification by Subsampling and Group Privacy Amplification
Jan Schuchardt, Mihail Stoian*, Arthur Kosmala*, Stephan Günnemann
Conference on Neural Information Processing Systems (NeurIPS), 2024
[PDF]
Provable Adversarial Robustness for Group Equivariant Tasks: Graphs, Point Clouds, Molecules, and More
Jan Schuchardt, Yan Scholten, Stephan Günnemann
Conference on Neural Information Processing Systems (NeurIPS), 2023
[PDF]
Hierarchical Randomized Smoothing
Yan Scholten, Jan Schuchardt, Aleksandar Bojchevski, Stephan Günnemann
Conference on Neural Information Processing Systems (NeurIPS), 2023
[PDF]
Localized Randomized Smoothing for Collective Robustness Certification
(selected for spotlight presentation)
Jan Schuchardt*, Tom Wollschläger*, Aleksandar Bojchevski, Stephan Günnemann
International Conference on Learning Representations (ICLR), 2023
[PDF]
Invariance-Aware Randomized Smoothing Certificates
Jan Schuchardt, Stephan Günnemann
Conference on Neural Information Processing Systems (NeurIPS), 2022
[PDF]
Randomized Message-Interception Smoothing: Gray-box Certificates for Graph Neural Networks
Yan Scholten, Jan Schuchardt, Simon Geisler, Aleksandar Bojchevski, Stephan Günnemann
Conference on Neural Information Processing Systems (NeurIPS), 2022
[PDF]
Generalization of Neural Combinatorial Solvers Through the Lens of Adversarial Robustness
Simon Geisler & Johanna Sommer, Jan Schuchardt, Aleksandar Bojchevski, Stephan Günnemann
International Conference on Learning Representations (ICLR), 2022
[PDF]
Collective Robustness Certificates: Exploiting Interdependence in Graph Neural Networks
Jan Schuchardt, Aleksandar Bojchevski, Johannes Gasteiger, Stephan Günnemann
International Conference on Learning Representations (ICLR), 2021
[PDF]
Workshop papers
Fast Proxies for LLM Robustness Evaluation
Tim Beyer, Jan Schuchardt, Leo Schwinn, Stephan Günnemann
Workshop on Building Trust in LLMs and LLM Applications
International Conference on Learning Representations (ICLR), 2025
[PDF]
Training Differentially Private Graph Neural Networks with Random Walk Sampling
Morgane Ayle, Jan Schuchardt, Lukas Gosch, Daniel Zügner, Stephan Günnemann
Workshop on Trustworthy and Socially Responsible Machine Learning
Conference on Neural Information Processing Systems (NeurIPS), 2022
[PDF]
Research Internships
- 2024: Research Scientist Intern, Morgan Stanley, New York, USA
Education
- 2020: Master's thesis: Collective Robustness Certificates
- 2018 - 2020: M.Sc. Computer Science, Technical University of Munich
- 2018: Bachelor's thesis: Reinforcement Learning for Adaptation in Evolutionary Computation
- 2015 - 2018: B.Sc. Computer Science, Technical University of Munich