Machine Learning in Medical Imaging
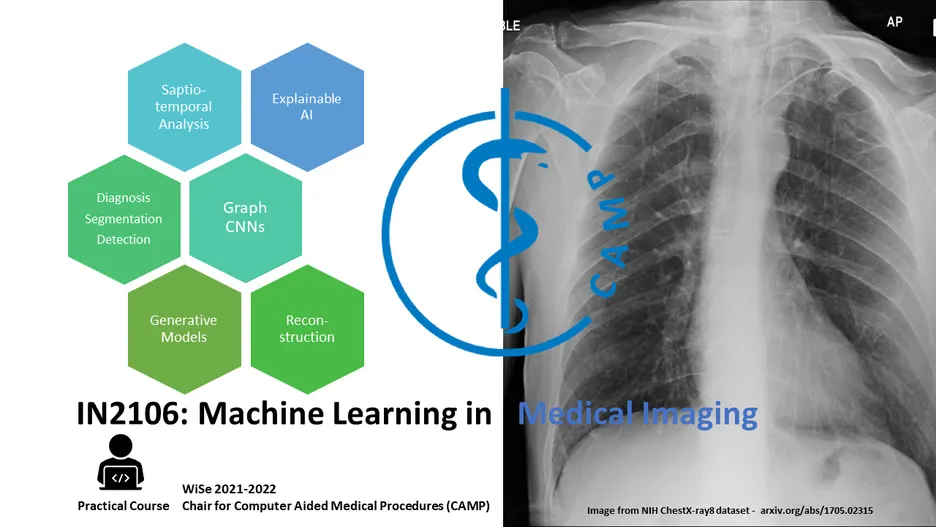
Overview
- The aim of the course is to provide the students with notions about various machine learning techniques. The course is mainly defined by a project.
- The topics of the projects will be distributed at the beginning of the semester. Each topic will be supervised by a different person. The projects are to be realized by couples.
- The preliminary meeting is scheduled for July 5th, from 14:00 to 14:30, with the following Zoom link:
https://tum-conf.zoom.us/j/63320903512?pwd=WEljYUtYUERHSFhzV1d1N2Iwd2JOZz09
Registration
- Registration must be done through TUM Matching Platform (please pay attention to the Deadlines)
- In order to increase your priority, please also apply via our own Registration system.
- The maximum number of participants: 24.
Master Practical Course - Machine Learning in Medical Imaging (IN2106, IN4142)
Lecturer (assistant) | |
---|---|
Number | 0000001192 |
Type | practical training |
Duration | 6 SWS |
Term | Wintersemester 2023/24 |
Language of instruction | English |
Position within curricula | See TUMonline |
Dates | See TUMonline |
Dates
- 17.10.2023 16:00-18:00 03.13.010, Seminarraum
- 24.10.2023 16:00-18:00 03.13.010, Seminarraum
- 31.10.2023 16:00-18:00 03.13.010, Seminarraum
- 07.11.2023 16:00-18:00 03.13.010, Seminarraum
- 14.11.2023 16:00-18:00 03.13.010, Seminarraum
- 21.11.2023 16:00-18:00 03.13.010, Seminarraum
- 28.11.2023 16:00-18:00 03.13.010, Seminarraum
- 05.12.2023 16:00-18:00 03.13.010, Seminarraum
- 12.12.2023 16:00-18:00 03.13.010, Seminarraum
- 19.12.2023 16:00-18:00 03.13.010, Seminarraum
- 09.01.2024 16:00-18:00 03.13.010, Seminarraum
- 16.01.2024 16:00-18:00 03.13.010, Seminarraum
- 23.01.2024 16:00-18:00 03.13.010, Seminarraum
- 30.01.2024 16:00-18:00 03.13.010, Seminarraum
- 06.02.2024 16:00-18:00 03.13.010, Seminarraum
Admission information
See TUMonline
Note: For registration, you have to be identified in TUMonline as a student. Interested students should attend the preliminary meeting. This semester we will have a joint presentation of the MLMI and DLMA courses offered, on Wednesday, July 5th, 2023, from 14:00 to 15:00 hrs with the following agenda: Machine Learning in Medical Imaging (MLMI): 14:00 hrs. - 14:30 hrs. Deep Learning for Medical Applications (DLMA): 14:30 hrs. - 15:00 hrs. Feel free to attend all the talks of your interest. The sessions will be conducted in Zoom: https://tum-conf.zoom.us/j/63320903512?pwd=WEljYUtYUERHSFhzV1d1N2Iwd2JOZz09 Please find the latest information on the course Wiki: https://wiki.tum.de/display/mlmi/MLMI+Winter+2023-2024
Note: For registration, you have to be identified in TUMonline as a student. Interested students should attend the preliminary meeting. This semester we will have a joint presentation of the MLMI and DLMA courses offered, on Wednesday, July 5th, 2023, from 14:00 to 15:00 hrs with the following agenda: Machine Learning in Medical Imaging (MLMI): 14:00 hrs. - 14:30 hrs. Deep Learning for Medical Applications (DLMA): 14:30 hrs. - 15:00 hrs. Feel free to attend all the talks of your interest. The sessions will be conducted in Zoom: https://tum-conf.zoom.us/j/63320903512?pwd=WEljYUtYUERHSFhzV1d1N2Iwd2JOZz09 Please find the latest information on the course Wiki: https://wiki.tum.de/display/mlmi/MLMI+Winter+2023-2024
Description
This Master-Praktikum will consist in: (1) a few introductory lectures on machine learning and its application in different medical imaging applications, (2) a few exercises to apply different learning approaches in toy examples and (3), a machine learning project with a real medical application.
Prerequisites
* Strong knowledge of Python
* Knowledge of main concepts of object oriented programming, basic software engineering.
* Knowledge of deep learning (should have taken an introductory course level)
* Knowledge of main concepts of object oriented programming, basic software engineering.
* Knowledge of deep learning (should have taken an introductory course level)
Examination
Exercises + Final Project