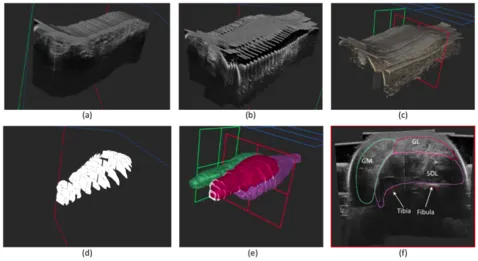
d) Sparse annotations e) Interpolations f) Cross-sectional view Solius (SOL), Gastrocnemius Lateralis (GL), Gastrocnemius Medialis (GM).
Open-Access Dataset for the Low-limb-Leg multilabel dataset
Motivation
Ultrasound (US) imaging is essential in diagnosing and managing musculoskeletal conditions, yet traditional 2D freehand ultrasound suffers from considerable variability due to operator dependency. This variability can impact diagnostic accuracy and treatment consistency. To overcome these limitations, the integration of 3D ultrasound technology, particularly when combined with advanced tracking systems, offers a significant advancement. 3D ultrasound creates comprehensive volumetric datasets, providing a more detailed and accurate representation of anatomical structures. This is especially beneficial in areas where understanding the spatial relationships between tissues is crucial for accurate diagnosis and effective treatment.
An innovative approach to harnessing the benefits of 3D imaging involves using freehand 2D ultrasound with tracking technology. This method transforms existing 2D ultrasound equipment into a system capable of generating 3D volumes by reconstructing a 3D image from a series of 2D scans, tracked in space and time. This cost-effective solution is gaining popularity for its adaptability and ease of use. Concurrently, the integration of machine learning (ML) algorithms with 3D ultrasound data can significantly enhance the identification and segmentation of anatomical features and pathologies. However, the effectiveness of ML depends on the availability of extensive, annotated 3D ultrasound datasets. Therefore, creating and sharing these datasets, especially those with high-resolution derived from tracked freehand 2D ultrasound, is vital for developing more accurate and reliable diagnostic and treatment methods that combine 3D imaging and AI technologies.
When using this dataset please refer to the paper:
[1] Ultrasound Segmentation Analysis via Distinct and Completed Anatomical Borders. Duque, V. G., Marquardt, A., Velikova, Y., Lacourpaille, L., Nordez, A., Crouzier, M., Lee, H. J., Mateus, D., & Navab, N. (Year). IJCARS Journal, - July 29, 2022 - https://doi.org/10.1371/journal.pone.0268550
Dataset Description
The dataset assembles pairs of Ultrasound volumes and 3-labels muscles of the low-limb leg from 44 healthy volunteers, aged between 18 and 45 years, with an average height of 173±11 cm and body mass of 64.3±12.4 kg.Three-dimensional arrays are in MetaImage Medical Format (MHA), with an average voxel grid of 564×632×1443 (±49×38×207), with an isotropic voxel spacing of about 0.276993 mm³ (±0.015 mm³). The ultrasound imaging utilized a 40mm linear VERMON probe with a frequency range of 2-10 MHz, and an Aixplorer, Supersonic Imagine Ultrasound machine. The tracking of the ultrasound probe was meticulously performed with a 6-camera Optitrack system. During the scans, participants were positioned prone with their leg in a custom-made bath, ensuring minimal pressure influence on the measurements. Multiple parallel sweeps, ranging from the knee to the ankle and recorded every 5 mm in low-speed mode, were conducted. The resulting high-resolution 3D ultrasound volumes were compounded using the tracking matrices of the probe, offering an unprecedented level of detail and accuracy in musculoskeletal imaging. The annotations, carried out by two double-blinded experts, focused on evaluating intra-operative volumetric error, which was found to be as low as 4%.
- Ultrasound volumes were generated using a Voxel-Based Method reconstruction algorithm,
- Labels represent four different muscle types with integer values {0,100,150,200} corresponding to the background, Soleus (SOL), Gastrocnemius Medialis (GM), and Gastrocnemius Lateralis (GL).
Dataset Access
The Leg-3D-US datasets can be downloaded with the following links:
Test dataset: https://www.campar.in.tum.de/public_datasets/2024_IPCAI_Vanessa/leg_test_data.zip
Validation dataset: https://www.campar.in.tum.de/public_datasets/2024_IPCAI_Vanessa/leg_validation_data.zip
Train dataset: https://www.campar.in.tum.de/public_datasets/2024_IPCAI_Vanessa/leg_train_data.zip
Github code with the datasheet of the dataset: https://github.com/Al3xand1a/segmentation-border-analysis
License
This dataset is licensed under a GNU General Public License (GPL). It requires that any derivative works also be open-source and distributed under the same terms. It ensures that any modifications to it remain open-source.
Potential technical applications
This dataset can be used for many deep-learning tasks, for example, for network label variability evaluation or to create and analyze the way we evaluate segmentation performance as we did in Duque et al. 2024 IPCAI publication. Data can also serve to pre-train networks that will be fine-tuned in other ultrasound datasets. Additionally, the size of this dataset, when treated as 2D images slicing the 3D volumes, makes it interesting for labeling propagation.
Acknowledgements
We would like to thank volunteers, as well, as ImFusion GmbH for providing us with software for visualizing the data (visualizations of the exemplary images are obtained using their "ImFusion Suite").