Neural Volume Compression and Rendering
Sebastian Weiss1, Philipp Hermüller1, Rüdiger Westermann1
1 Chair for Computer Graphics and Visualization, Technical University of Munich, Germany
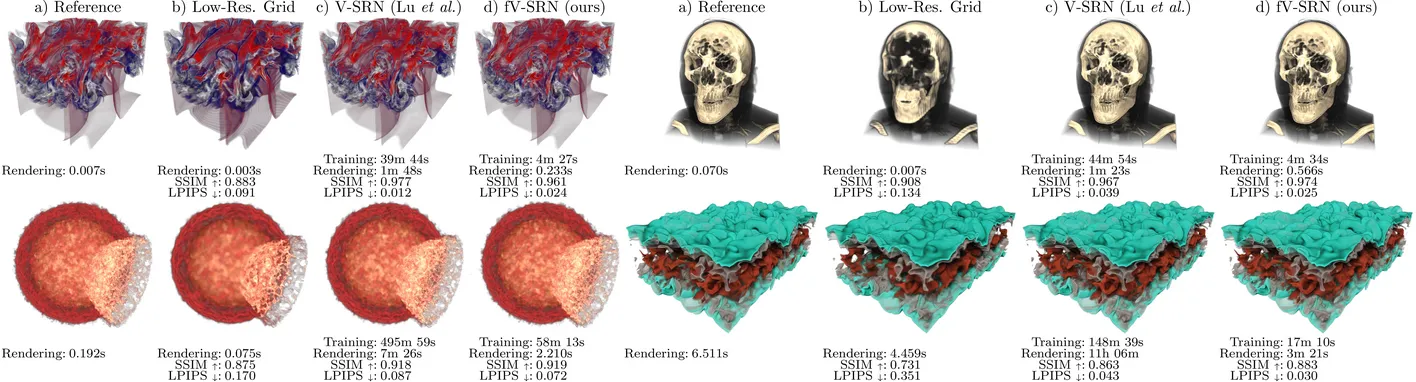
Abstract
Despite the potential of neural scene representations to effectively compress 3D scalar fields at high reconstruction quality, the computational complexity of the training and data reconstruction step using scene representation networks limits their use
in practical applications. In this paper, we analyze whether scene representation networks can be modified to reduce these limitations and whether such architectures can also be used for temporal reconstruction tasks. We propose a novel design of scene representation networks using GPU tensor cores to integrate the reconstruction seamlessly into on-chip raytracing kernels, and compare the quality and performance of this network to alternative network- and non-network-based compression schemes. The results indicate competitive quality of our design at high compression rates, and significantly faster decoding times and lower memory consumption during data reconstruction. We investigate how density gradients can be computed using the network and show an extension where density, gradient and curvature are predicted jointly. As an alternative to spatial super-resolution approaches for time-varying fields, we propose a solution that builds upon latent-space interpolation to enable random access reconstruction at arbitrary granularity. We summarize our findings in the form of an assessment of the strengths and limitations of scene representation networks for compression domain volume rendering, and outline future research directions.
Associated publications
Fast Neural Representations for Direct Volume Rendering
Sebastian Weiss, Philipp Hermüller, and Rüdiger Westermann
Computer Graphics Forum, 2022
[Paper] [BIB] [Code]